Math and science::INF ML AI
Belief networks: independence examples
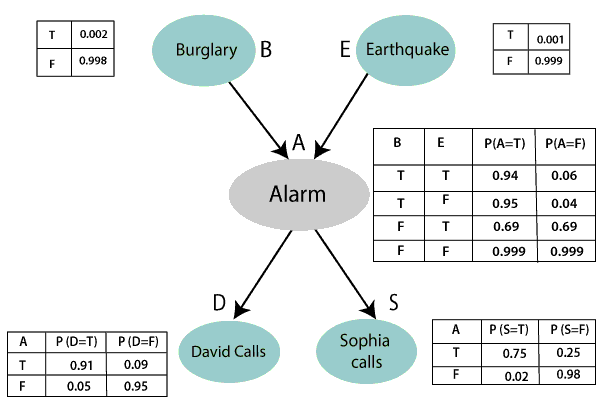
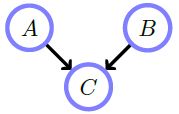
1 a) Marginalizing over makes and independent. In other words, and are (unconditionally) independent . In the absence of any information about the effect of we retain this belief.
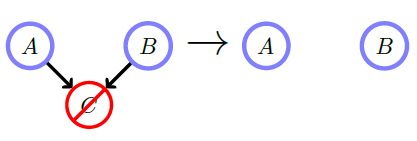
1 b) Conditioning on makes A and B (graphically) dependent . Although the causes are a priori independent, knowing the effect can tell us something about how the causes colluded to bring about the effect observed.
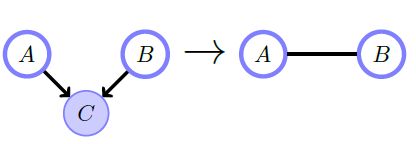
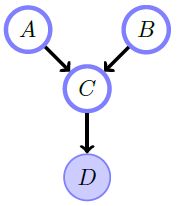
2. Conditioning on , a descendent of a collider makes and (graphically) dependent.
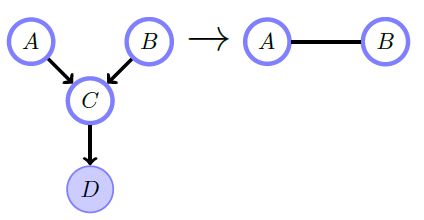
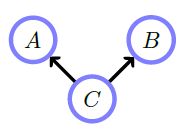
3 a) . Here there is a 'cause' and independent 'effects' and .
3 b) Marginalizing over makes and (graphically) dependent. . Although we don't know the 'cause', the 'effects' will nevertheless be dependent.
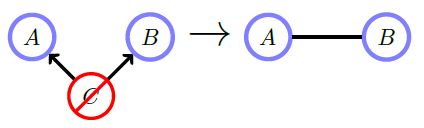
3 c) Conditioning on makes and independent. . If you know the 'cause' , you know everything about how each effect occurs, independent of the other effect. This is also true for reversing the arrow from to —in this case, would 'cause' and then would 'cause' . Conditioning on blocks the ability of to influence .
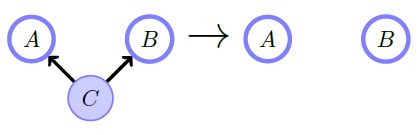
Finally, these following graphs all express the same conditional independence assumptions.

Source
Bayesian Reasoning and Machine LearningDavid Barber
p42-43