Math and science::INF ML AI
Chi-squared distribution
David MacKay motivates (in passing) the chi-squared distribution through exercise 2.5.
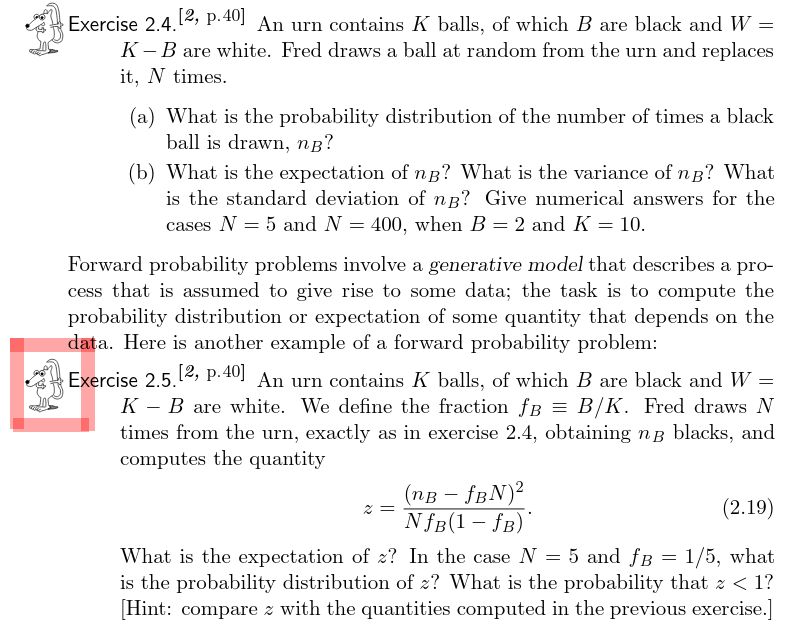
The solution is on the reverse side. Dummy cloze
Recognize that \( z \) is a function of the of a random variable \( n_B \) which is in turn a sum of independent Bernouilli random variables. \( z \) can be thought of as trying to measure how many standard devations away from the mean \( n_B \) is.
The official chi-squared distribution is specific to the case where \( n_B \) is normally distributed with some mean and variance.